In today’s data-driven world, organizations are increasingly relying on data analytics to gain valuable insights and make informed decisions. Data Lakehouse, a powerful data storage and analytics framework, offers a scalable and cost-effective approach to manage and analyze vast amounts of data. In this blog post, we will explore the key features and benefits of a Data Lakehouse and how it empowers organizations to maximize their data analytics potential.
What Is A Data Lakehouse
A Data Lakehouse is a modern data architecture that addresses some limitations and challenges associated with traditional Data Lakes and Data Warehouses. It combines the advantages of each, aiming to provide a unified and scalable platform for storing, processing, and analyzing data, offering the best of both worlds to end users.
Both Data Lakes and Data Warehouses serve specific purposes and cannot alone handle the complexities of modern data environments.
A Data Lake is a storage repository that can handle large volumes of structured, semi-structured, and unstructured data. Data is stored in its raw and original format, without a predefined schema.
A Data Warehouse is a data storage and processing system that specializes in storing structured data. It utilizes a predefined schema and is highly optimized for efficient querying and analytics processes.
In a Data Lakehouse architecture, the Data Lake serves as the central repository for storing large volumes of raw data. The data is then transformed, curated, and organized into a structured format within the Data Lakehouse.
Once the data is organized and structured, it can be accessed by analytics tools and platforms for querying, reporting, and machine learning.
The Data Lakehouse architecture enables both batch and real-time processing, combining the scalability and cost-effectiveness of a Data Lake with the performance optimizations and query capabilities of a Data Warehouse.
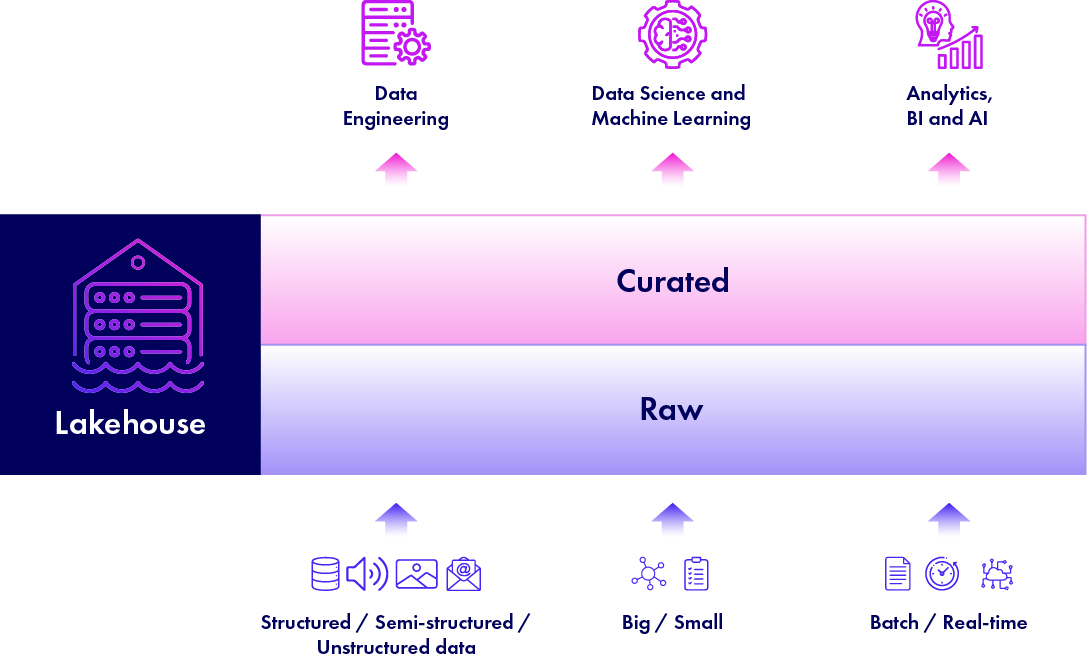
Figure 1: Lakehouse architecture (@proskale.com)
Features of a Data Lakehouse
- Unified data storage
- Schema enforcement
- Data engineering and data science
- Time travel analysis
- Real-time insights
- IoT data processing
- Streaming data processing
- Concurrent read and write (ACID compatibility of transactions)
- Customer 360
- Scalability and performance
- Cost effectiveness
- Integration with multiple cloud services
- Security
- Open and standardized storage formats
- Separation of storage and processing
- Advanced analytics
Concluding Remarks
To summarize, a Data Lakehouse presents a robust and compelling solution that harnesses the strengths of both Data Lakes and Data Warehouses. It seamlessly integrates storage, processing, and analytics capabilities, enabling organizations to achieve smooth data integration, advanced analytics, and real-time insights. With its versatility in handling structured and unstructured data, Data Lakehouse empowers organizations to dismantle data silos and democratize data access, fostering a culture of collaboration and data-driven decision-making.
As organizations continue to realize the significance of their data assets, embracing a Data Lakehouse architecture becomes an essential strategic move. By adopting the Data Lakehouse, organizations embark on a transformative journey that unlocks the true potential of their data. This enables them to make well-informed decisions, foster innovation, and gain a competitive advantage in the digital era.
proSkale has developed several accelerators for building and managing cloud-based solutions, including Data Lakehouse for three major cloud service providers – Amazon AWS, Microsoft Azure, and Google Cloud. All three provide a framework for Data Lakehouse and we will explore each further in future posts. Our large pool of skilled and trained cloud data engineers and analysts have helped many of our clients progress their digital transformation journeys and, using proSkale’s cloud and data analytics solutions, have stood up a Data Lakehouse architecture within a matter of weeks. Contact us today to discuss how we can rapidly help your modernize your data and supporting applications.
References:
https://proskale.com/3-minute-overview-the-azure-modern-data-platform/
https://proskale.com/proskales-cloud-migration-strategy/
https://proskale.com/drastically-reduce-etl-overhead-with-low-code-solutions/
https://proskale.com/databricks-vs-synapse/
https://techcommunity.microsoft.com/t5/azure-synapse-analytics-blog/building-the-lakehouse-implementing-a-data-lake-strategy-with/ba-p/3612291
https://www.forbes.com/sites/bernardmarr/2022/01/18/what-is-a-data-lakehouse-a-super-simple-explanation-for-anyone/?sh=7f5796126088
https://www.dremio.com/blog/what-is-a-data-lakehouse/
https://www.oracle.com/big-data/what-is-data-lakehouse/
https://services.google.com/fh/files/misc/building-a-data-lakehouse.pdf
https://aws.amazon.com/blogs/big-data/build-a-lake-house-architecture-on-aws/
https://learn.microsoft.com/en-us/azure/architecture/example-scenario/analytics/secure-data-lakehouse-synapse
https://learn.microsoft.com/en-us/azure/databricks/lakehouse-architecture/
https://docs.delta.io/
https://databricks.com/blog
https://www.forbes.com/sites/forbestechcouncil/2022/08/24/evolving-big-data-strategies-with-data-lakehouses-and-data-mesh/?sh=1c43f1525fef